Projections of potential policy impact and the transition to electric vehicles are complicated for medium- and heavy-duty vehicles. Predicting accurate outcomes may be difficult, but possible, if the models incorporate market dynamics comprehensively.
The widespread adoption of electric vehicles could reduce greenhouse gas emissions substantially from the US transportation sector, thus improving air quality and public health while helping achieve climate goals. Incentives and regulations in the transportation sector thus aim to increase the adoption of electric vehicles. For example, in April, the US Environmental Protection Agency took notable steps in the pursuit of cleaner transportation, announcing final greenhouse gas emission standards for heavy-duty trucks. Additionally, states are rolling out their own initiatives, with California leading through its Zero-Emission Vehicle mandates for light-duty vehicles and its introduction of the Advanced Clean Fleets and Advanced Clean Trucks regulations for the heavier vehicle classes.
Yet, the transition to electrification for medium- and heavy-duty trucks is more complex than for passenger vehicles, requiring greater infrastructural investments and more complicated decisions that involve vehicle fleets rather than individual vehicles. Accurate models and forecasting in this sector are essential to understanding market transitions, determining the appropriate stringency of regulatory standards, and ensuring compliance with international climate pledges. In this blog post, we explore such complexities, emphasizing the importance of accurate modeling—and noting that failure to address these intricacies may lead to overly optimistic expectations about the costs and the efficacy of a given policy.
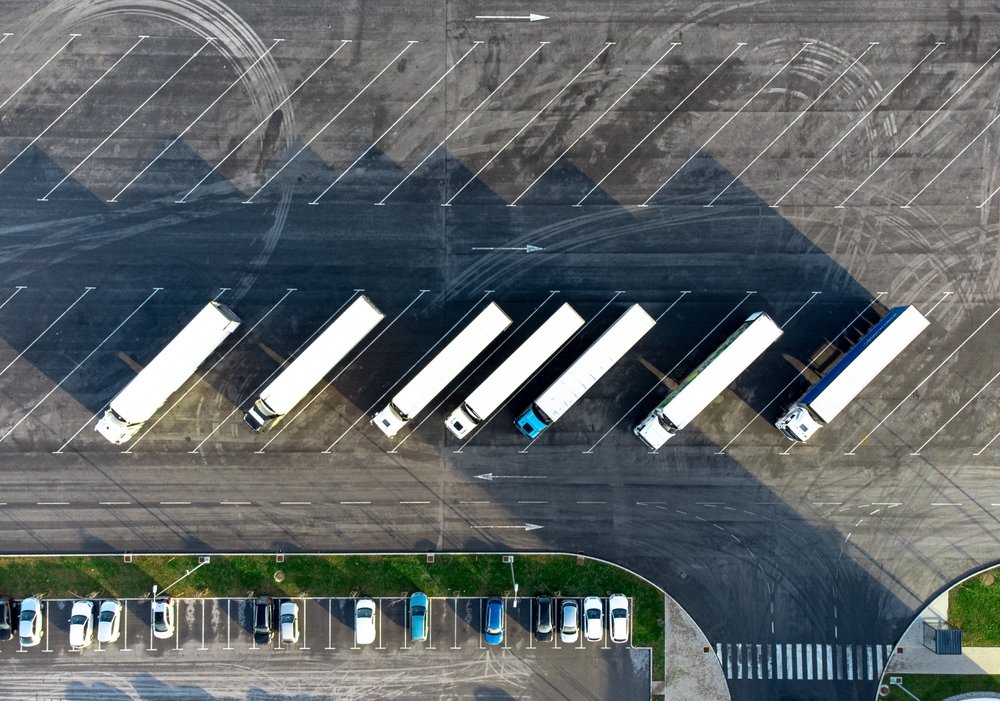
Data from 2022 indicate that medium- and heavy-duty electric trucks constituted only 0.4 percent of registrations for all new trucks in the United States. Various hurdles may hinder the widespread adoption of medium- and heavy-duty electric vehicles, including the high up-front cost of electric trucks and buses, the high cost and low availability of charging infrastructure, and complexities surrounding electricity tariffs. Regulatory impact analyses that use models like the MOtor Vehicle Emission Simulator, EMission FACtor model, or total cost of ownership–based models often fail to fully capture some of these hurdles, particularly those that require changes in logistics, behavior, or learning by fleet managers.
For example, the EMission FACtor model relies on sales forecasts from the US Energy Information Administration’s Annual Energy Outlook to assess the impact of regulatory measures, but the model doesn’t account for how these regulations influence decisions for fleet purchases. Consider California’s Advanced Clean Trucks regulation, which mandates that manufacturers sell a certain percentage of zero-emission vehicles. If there are significant barriers to electric vehicle adoption, the model will likely yield overly optimistic projections for new electric vehicle sales.
While the total cost of ownership–based models effectively capture visible hurdles, such as the up-front price of vehicles and chargers, they do not include less obvious hurdles such as fleet owners’ preferences for specific vehicle features, nor transition costs such as those that arise from having to navigate complex electricity tariffs. By not incorporating the preferences of fleet owners or transition costs in their models, regulatory agencies risk underestimating the costs or overestimating the efficacy of a regulation.
Furthermore, total cost of ownership–based models do not adjust new and used vehicle prices and sales forecasts in response to stringent regulations. Because regulations target only new trucks, these policies may contribute to increased up-front costs to purchase these vehicles (and potentially affect the price of vehicles in the used market); thus, these models would fail to consider the very real possibility that fleets might continue using older vehicles for extended periods. In fact, historical data reveal a consistent increase in truck lifespans and a decline in their scrappage rates.
To explore this issue more directly, we analyzed vehicle registration records from five different years: 2002, 2007, 2012, 2017, and 2022. Figure 1 shows the five-year scrappage rates for certain classes of medium- and heavy-duty vehicles, broken down by vehicle age for these years. Each line represents a different five-year interval. For each age group, the values show the percentage of trucks that were taken off the road within that five-year interval. For example, the yellow curve indicates that 10 percent of 50-year-old trucks registered in 2017 were taken off the road before 2022. Moreover, the end points of the curves on the x-axis indicate the age of the oldest vehicles that were registered in each year.
Figure 1. Proportion of Aging Trucks Taken Off the Road within Five Years of Last Registration
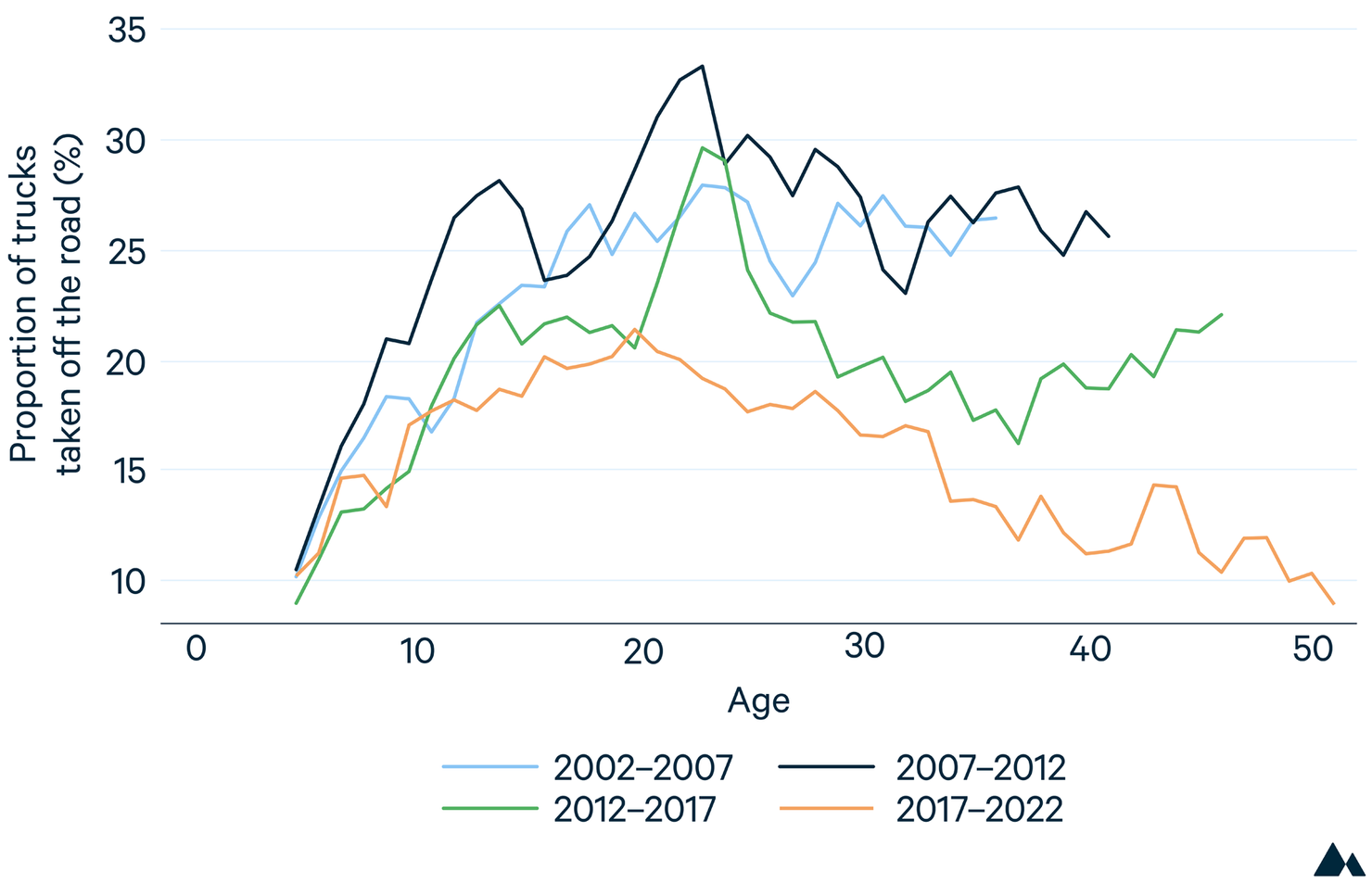
Figure 1 shows that the likelihood of a truck being taken off the road has decreased notably over time. As a result, the oldest vehicles registered in the United States were 36 years old in 2002, which progressively aged to 41 years in 2007, 46 years in 2012, and as old as 51 years in 2017.
This trend may be attributed, in part, to regulations that allow older trucks to remain in operation without adhering to newer emissions standards, potentially reducing the financial incentive to invest in newer, more environmentally friendly models. By omitting information about scrappage decisions by fleet owners in their models, agencies risk overestimating the benefits of regulations.
Moreover, additional market dynamics, such as market power held by one or a few truck manufacturers, can maintain high prices, further hindering the adoption of new technologies by vehicle fleets. These dynamics are especially true at the beginning of a market transition, when a recognizable brand dominates the market until higher-quality products become available and gain market share.
This challenge of persistent high prices necessitates a more sophisticated approach to modeling outcomes that is grounded in game theory, which can adequately incorporate market structure into analyses. A 2023 analysis from Resources for the Future underscores this issue by scrutinizing data on truck characteristics from 2021 to 2023. The report shows that, for large vehicles, the price gap between conventional and electric trucks markedly exceeds the intrinsic cost of the battery (Figure 2). In the case of the largest vehicles (the heavy-duty category), the price differential is about three times the cost of the battery. This discrepancy can be partly attributed to low economies of scale and limited competition within the market, factors that exacerbate the challenge of transitioning fleets to more sustainable options.
Figure 2. Price Differential and Battery Cost across Vehicle Type
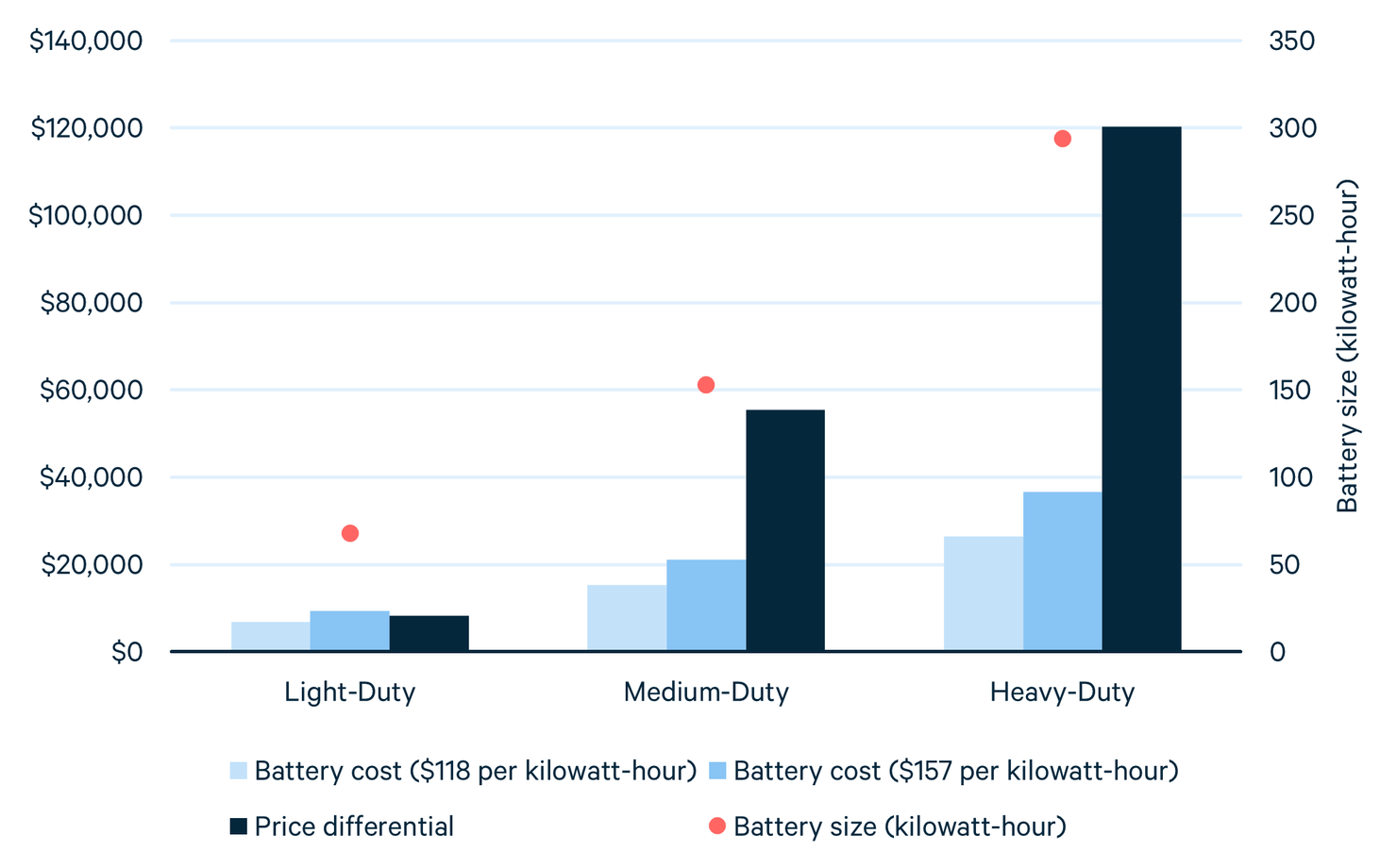
This figure originally appeared in a May 2023 blog post about the high cost of electric trucks.
Rather than estimating price markups based on game-theoretic foundations and actual data, agency analyses often simply assume the size of the markup. Accurate assessment of these market conditions is crucial for understanding how regulations and incentives influence vehicle prices. For example, the Inflation Reduction Act’s purchase subsidies for electric vehicles and charging stations may not be fully passed through to the buyer. This is because, in markets with low competition, incentives that are designed to encourage electric vehicle adoption may inadvertently enable manufacturers to increase pre-incentive prices. This dynamic may, in the end, delay the transition to electric vehicles. Failing to accurately capture the market structure can lead to overestimated cost reductions for fleets.
The need for advanced modeling of this sector is readily apparent. The complexity of the transition to electric fleets, and the myriad policies implemented to support this transition, call for the development and use of a comprehensive econometric, data-based analysis of demand and supply in the medium- and heavy-duty vehicle sector that captures preferences for vehicle characteristics, market dynamics that affect price, and real-world costs of electrification. Without this type of modeling, predictions about the costs and efficacy of existing policies may be overly optimistic.