Resources for the Future (RFF) is looking to grow its team of researchers, and our latest additions are Fellows Yanjun (Penny) Liao and Hannah Druckenmiller. These two scholars now have nearly a year under their belts at RFF—and one even has a new baby. Both research fellows spoke about their backgrounds and their latest research with podcast hosts Daniel Raimi and Kristin Hayes on Resources Radio, a weekly podcast produced by the Resources editorial team and RFF. These conversations with Penny and Hannah are transcribed here, serving as introductions to some of the more recent members of RFF’s research team.
These interviews were broadcast in August 2021. The transcripts of these conversations have been edited for length and clarity.

Yanjun (Penny) Liao
Risks and Rewards in Homeownership and Flood Insurance
Penny Liao, a scholar of behavioral and market responses to environmental risk, joined Resources for the Future (RFF) as a fellow in August last year. On Resources Radio, Liao elaborated on her research about how a household decides to purchase flood insurance, finding that homeowners with more home equity are especially likely to purchase flood insurance because they have stronger incentive to avoid defaulting on their mortgage, while households with highly leveraged mortgages might not fully account for their flood risks.
Listen to the Podcast
Kristin Hayes: Can you tell our listeners about the path that brought you to this point?
Penny Liao: I actually started on this path to be an environmental economist by chance. I was an undergraduate in economics at the University of Hong Kong, and nobody in my university studied environmental economics. The field does not exist there. But I happened to know an environmental economist teaching at another university in Hong Kong, named Professor Bill Barron, and he was incredibly passionate about the field. As soon as he learned that I was an undergrad in econ, he immediately recommended two papers for me to read.
Those are “The Tragedy of the Commons” by Garrett Hardin and “The Problem of Social Cost” by Ronald Coase, and these papers blew my mind. They’re such seminal papers in the field, and they laid out very profound and powerful ideas in a very accessible way that I—as a second-year undergrad—could understand. I remember being so fascinated by the idea that you can apply an economic lens to environmental problems. That appeals to me a lot, because growing up in China, I’ve seen the tension between economic development and environmental quality. And environmental economics seems to have this potential for confronting this tension and finding a path forward.
So, this fascination has stuck with me ever since. I started working for an environmental and urban policy think tank in Hong Kong, called Civic Exchange. Then I went to the University of California, San Diego, to pursue a PhD in economics, specializing in environmental economics. When I completed my PhD, I was already working on climate and disaster impacts.
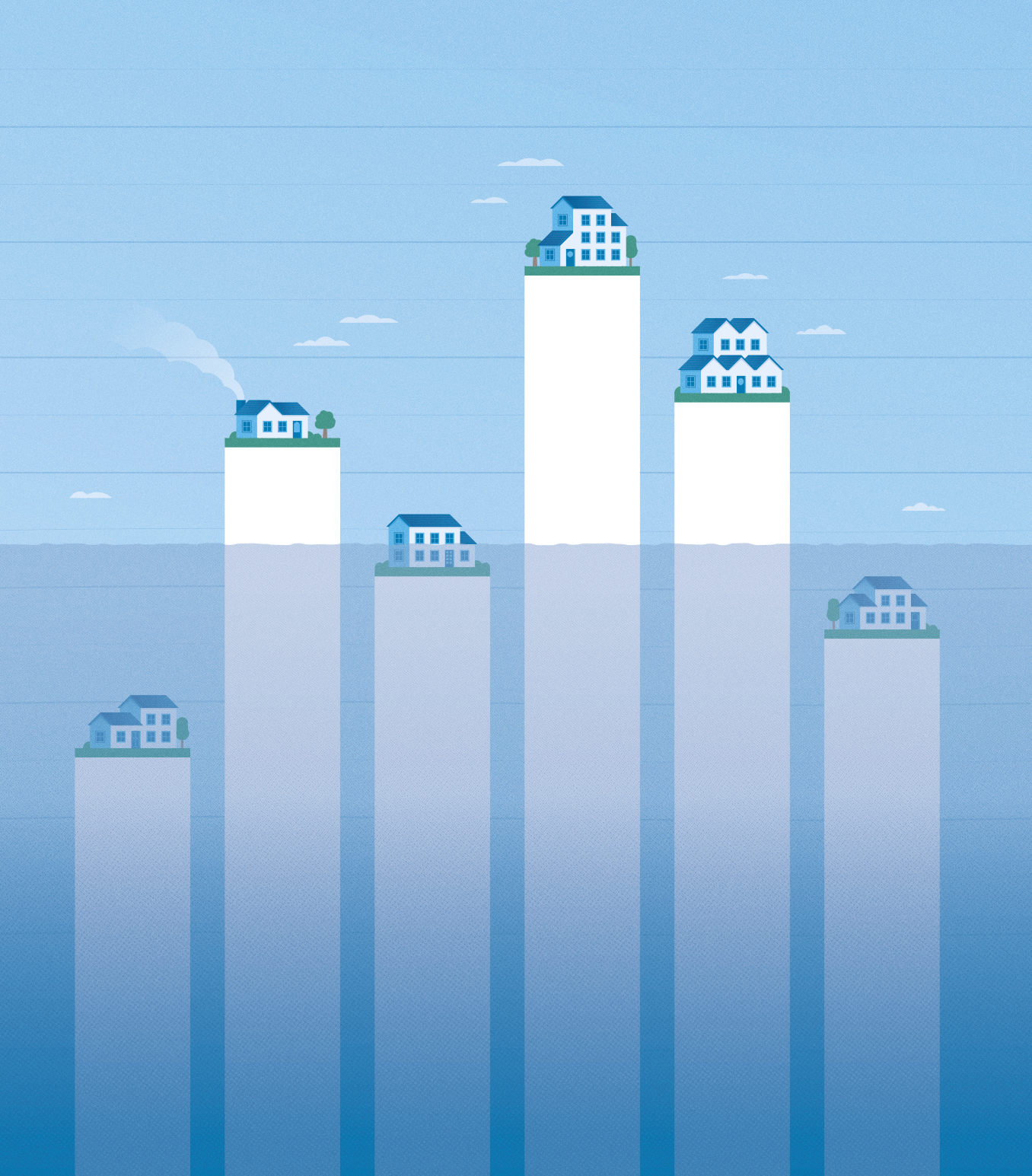
I then joined the Wharton Risk Center as a postdoctoral researcher. The Risk Center deals a lot with questions of risk—how people make decisions related to risks, how risks get diversified, and things like that. That’s when I started thinking about disaster risk management and adaptation more systematically.
I love the beginning of that anecdote, just because it reminds me how much of a difference an individual professor—or just a passionate person of any variety—can really make a difference in someone’s trajectory. But it sounds like you blazed your own path, in terms of following your passions and bringing things together in a way that wasn’t common.
PL: I was definitely lucky to have such good mentors along the way. I think that, during your formative years, running into these people really helps.
Can you say more about why you chose to focus on disasters, risks, and adaptation? Why did that capture your imagination?
PL: First of all, even without climate change, disaster risk is a very important and interesting topic to me. There are catastrophic events throughout human history that have destroyed the lives and livelihoods of so many people. We now have more advanced physical knowledge and modeling techniques than before, and we know that we can see this as a risk-management problem.
From a public policy point of view, I think it’s an important question: How do we build a robust system to reflect and diversify the risks, and to protect vulnerable populations from the realization of those risks? On top of that, we have climate change, which makes things much worse.
Flooding is a very expensive type of disaster, both in aggregate and for individual homeowners. In aggregate, it cost about $15 billion annually over the past decade.
Penny Liao
I think you spell it out really well in your question. The relative inaction on mitigation makes adaptation more important. That’s also the realization I’ve come to during graduate school, and I think there’s a lot of room for improvement when it comes to adaptation.
This summer, we’re seeing a record number of extreme weather events and disasters across the world. And it seems clear that the infrastructure in many places is not really well defined to handle such events. There are a lot of opportunities right now for governments to take note and be more prepared for unexpected events like this. It’s not just the governments—individuals, businesses, and other entities can also take actions to be prepared. There are a lot of open questions about whether we have the right incentive structure in place, for these agents to take the necessary adaptation measures.
At RFF, we focus on policy—and the policy levers are incredibly important, but you’re right to point out that adaptation is going to happen across a wide range of jurisdictions, including everything from the homeowner, to the insurance company, to the local government, state government, all the way up to the federal government. So, the range of questions and players is very wide. It makes sense that there’d be a lot that we still need to figure out.
You mentioned that you got your undergraduate education in Hong Kong, before coming to the United States, and that you grew up in China. Can I ask, for a global perspective, how you see the conversation around mitigation—and perhaps adaptation in particular—being different in a place like Hong Kong, compared to the United States? Are there different policy levers available? How does the conversation look?
PL: There are certainly both differences and similarities. First of all, in Hong Kong, climate change is not a politically charged issue, so I think the general public might not consider it a huge concern, compared to the economy, for example. But they do overwhelmingly support mitigating carbon emissions within Hong Kong. In terms of adaptation, Hong Kong is a predominantly urban environment: Most people live in an urban environment, and they have exposure to extreme heat and tropical cycles. The infrastructure there has more or less been adapted to such events, and management practices are trying to adapt to these risks.
But, going forward, it’s unclear whether the existing infrastructure is going to hold up in more extreme scenarios of heat and things like storm surges coming from sea level rise. I think that is actually universal to a lot of other places, as well, which are all facing uncertainty.
There is also a high level of inequality. I’m not sure I have seen enough discussion there about what this means, in terms of exposure to climate impacts by different groups. I think the equity concern is true both in Hong Kong and the United States. I think that there are definitely differences, but also a lot of similarities.
Interesting. Thank you for that context, with the trans-Pacific perspective there. You’re wrapping up some work on flood insurance and home equity, and you and your authors look at how “mortgage default may be a form of implicit insurance against disaster risk for leveraged households.” Can you explain that hypothesis and ground us in what you’re looking at?
PL: This paper is with Philip Mulder, who’s a graduate student at Wharton. In the paper, we’re interested in looking at flood insurance, but let me first give you some context.
Flooding is a very expensive type of disaster, both in aggregate and for individual homeowners. In aggregate, it cost about $15 billion annually over the past decade. For individuals, when their home is flooded, the damage can easily be tens of thousands of dollars. For example, in 2019, the average flood insurance claim was $52,000. In 2017, when there were particularly severe hurricanes, the average claim went to more than $90,000. These are flood insurance claims in the United States. They’re very high numbers for a normal household if they don’t have insurance. In the past, we’ve seen that flooding leads to higher rates of mortgage defaults and foreclosures.
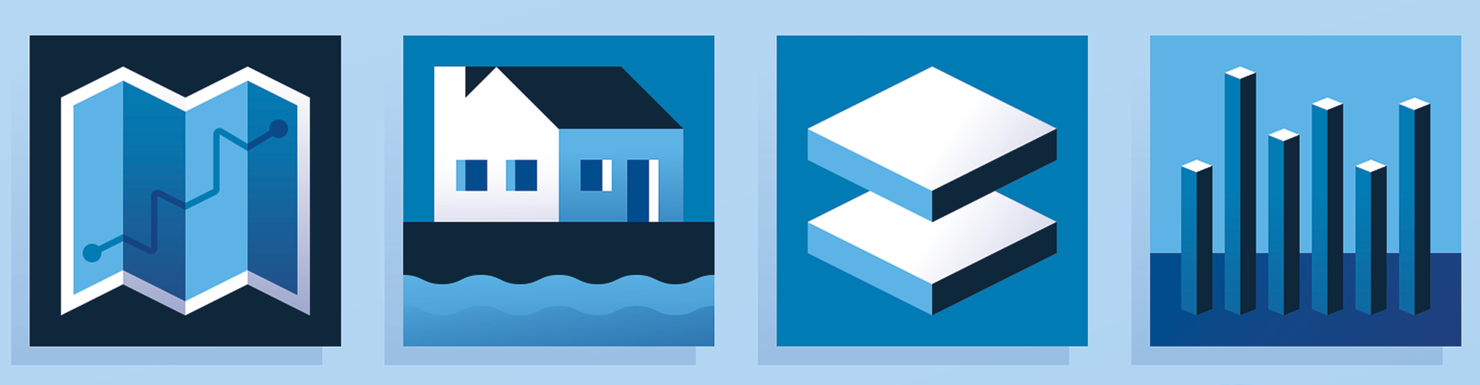
What that means is that some homeowners, instead of paying to repair the house out of pocket, would rather default on their mortgage and give up their home equity. This can be a rational choice when the homeowner has a low level of home equity but high flood damage. So, in this case, we can think of mortgage default as a kind of high-deductible insurance policy. The deductible is the home equity, but that’s all the homeowner’s going to lose when the flood damage goes beyond that.
The level of equity is the key here. If you have a lot of home equity, then you wouldn’t want to default, so you cannot rely on this implicit insurance. In that case, you would be better off buying formal flood insurance. So, the main prediction is that the more home equity you have, the more you’re willing to pay for flood insurance.
You took advantage of some previous fluctuations in housing market prices, to tease out this relationship between home equity and insurance uptake. Can you explain about the data sources that you used and how you found a moment in time when you felt like you’d be able to look at this question robustly?
PL: The main relationship we want to test for is that higher home equity increases flood insurance demand. For flood insurance data, the main source we used comes from the National Flood Insurance Program. This is a public program operated by the Federal Emergency Management Agency (FEMA), and it provides around 95 percent of all flood insurance in the United States. We’re capturing the vast majority of the market because, historically, private insurance companies are not willing to provide flood insurance coverage.
FEMA has published policy-level data in its open FEMA website. It’s a great data source for researchers who are interested in studying flood insurance, and that’s the data we used. We collected other data to supplement it as controls.
From a public policy point of view, I think it’s an important question: How do we build a robust system to reflect and diversify the risks, and to protect vulnerable populations from the realization of those risks?
Penny Liao
The most tricky thing in this research is a challenge in the research design, because home equity is correlated with other important factors in flood insurance demand, such as income, education, or risk attitudes. To identify the causal relationship, we needed to find something that drives home equity but not these other things. For that, we used sudden changes in housing prices during the housing boom and bust in the early 2000s. Around this time, we observed that there was a sudden price acceleration in some housing markets, but not others. In these housing markets, the price first grows smoothly. Then, between around 2003 and 2005, there was a sudden acceleration, where the price of new houses started growing much faster. This variation is likely to be speculative, and it’s independent from fundamental changes in economic and demographic conditions.
This drives large changes in home equity for many homeowners, but it does not change, for example, their underlying flood risk, or the expected cause of flooding for them, because they’re still living in the same buildings. This gives us a good opportunity to identify the causal effect of home equity on flood insurance demand, while holding these other factors constant.
We looked at all the metropolitan statistical areas across the entire United States.
What relationships did you discover? And how do you and your coauthors explain what you found?
PL: The first thing we see is that flood insurance take-up indeed increases more in high-boom markets when compared to low-boom markets. More importantly, we’re able to estimate how flood insurance take-up changes over time, in response to that shock, and trace that out over time. We find that the trajectory of insurance take-up correlates really well with the trajectory of housing prices in response to the same housing-market shocks. This suggests strongly to us that there is a direct relationship between the two, so we’re able to estimate this relationship directly.
We find that a 1 percent increase in housing prices leads to a 0.3 percent increase in flood insurance demand. To put this in context, this is twice the effect of a 1 percent drop in insurance premium. That’s a primary factor in insurance demand, so this suggests that there’s quite a substantial relationship.
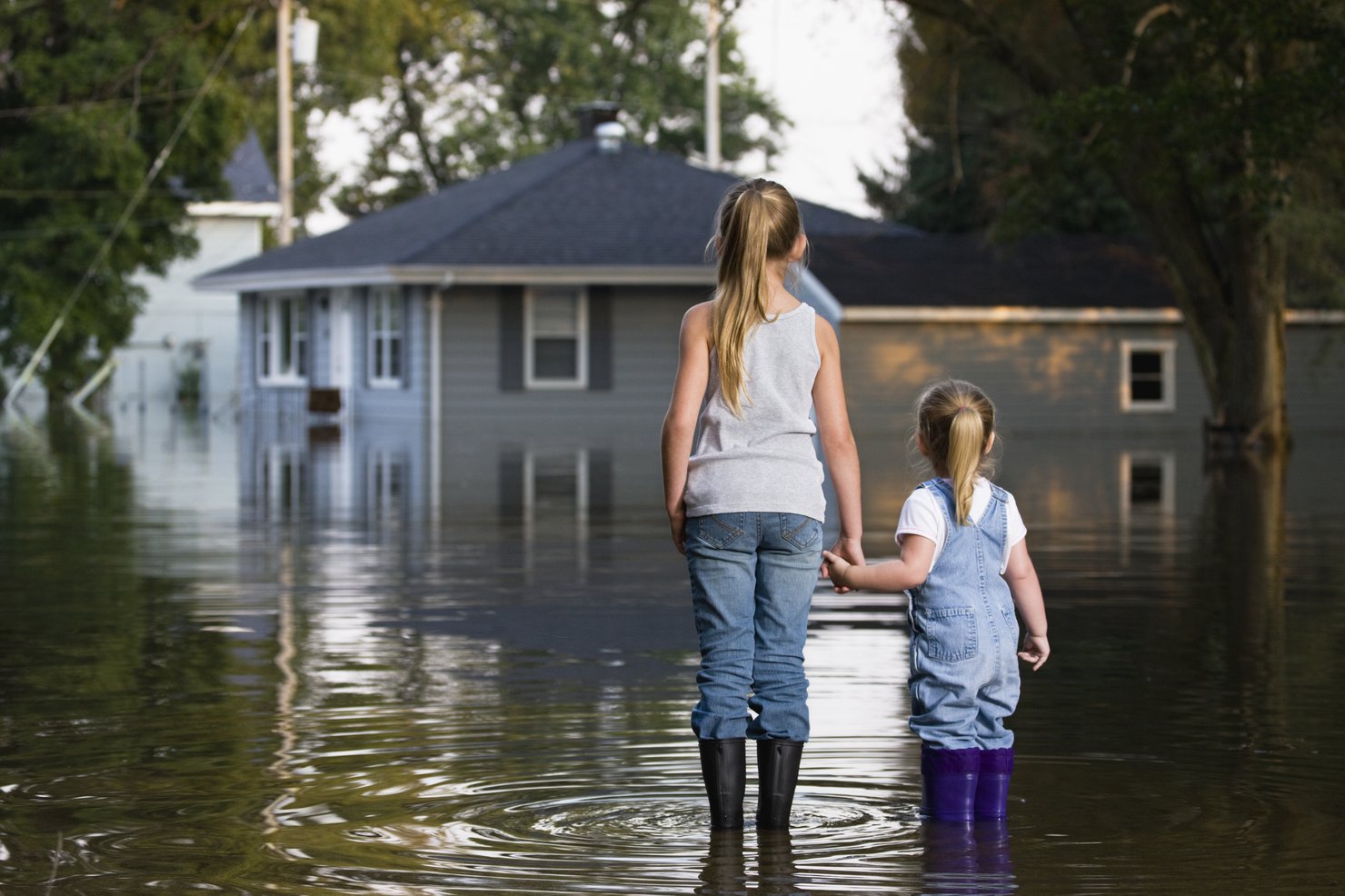
We also find other patterns that are very telling. For example, National Flood Insurance Program households that live in 100-year floodplains are mandated to buy flood insurance if they have a federally backed mortgage. But outside of the 100-year floodplains, there’s no mandate whatsoever—so, the decision is completely voluntary. We find that this is largely driven by those households living outside of the 100-year floodplains. So, we’re capturing this conscious decision: these are voluntary choices people are making.
To further establish that this is really driven by a mortgage default mechanism, we looked at how things were different across the metropolitan statistical areas with different foreclosure costs. By “foreclosure cost,” we mean things like, “How soon will I be evicted from the house? Would I be charged a large fee by the lender if I did?” This could vary across states based on their foreclosure laws. Some states require all the foreclosures to go to court, and these are called judicial requirements. When there’s a judicial requirement, it protects the borrower’s interest. We find that, indeed, in these places, the relationship between home equity and insurance demand is much stronger than the places without the judicial requirement. That also supports the mechanism.
It sounds like you did a tremendous amount of digging around with the information you had, and thank you for sharing those findings. How do you see all this affecting future decisionmaking? In other words, what would you want policymakers and other decisionmakers to take away from what you’ve done, as they’re designing future flood insurance programs or thinking about how to better protect homeowners in the future?
PL: The first implication from the findings is that homeowners with a highly leveraged mortgage do not fully internalize their flood risk. Instead, part of the risk is transferred to the lenders, but ultimately, a lot of these loans are securitized by government-sponsored enterprises (GSEs), such as Fannie Mae and Freddie Mac. Taxpayer dollars are on the line, and this is an implicit cross-subsidy to homeowners exposed to flood risk.
It leads to the second implication, which is that the implicit subsidy here can distort incentives by these homeowners to insure, which we have shown in the paper. It was similarly a factor in people’s incentive to take adaptation measures, such as floodproofing their homes.
We think that some possible solutions to address this problem of incentives is to focus on reflecting the risk in the mortgage system, especially for homes outside of the 100-year floodplains—as we find that’s where the effect is. The GSEs, for example, could consider pricing the risk they have taken on, such as charging a higher fee to securitize at-risk loans without insurance coverage. Alternatively, it’s worth considering expanding the flood insurance mandate to beyond the 100-year floodplains, because that 1 percent risk cutoff is pretty artificial. Homes outside that zone also are exposed to quite substantial levels of risk.
Taxpayer dollars are on the line, and this is an implicit cross-subsidy to homeowners exposed to flood risk.
Penny Liao
I’ve also mentioned the incentive to take adaptation measures. It’s also important for flood insurers to price in these risk-reduction measures as a way to encourage people to undertake them. These are things like receiving a discount when you have undertaken certain floodproofing measures.
Recently, we have seen some promising steps taken by different federal agencies, which I think are going in the right direction. For example, FEMA has come out with Risk Rating 2.0, which aims to provide more accurate risk-based pricing to insurers. The Federal Housing Finance Agency, which is the main regulator of the GSEs, issued a request for input on climate and disaster risk in April, which reflects them giving this issue real attention. So, I think it will be very interesting to see where these efforts take us; they could even be future research topics.
Penny, thank you for explaining all of that so clearly and for grounding us in the work you’re doing. What do you hope to work on as you start at RFF, building on what you’ve been doing in the past, as well as issues that you’ve been talking about with colleagues on staff? Are there things that you’re particularly excited about tackling in your ongoing research career?
PL: Yeah, definitely. I’m going to continue this line of work, in general, thinking more about how we handle risk—especially as climate change is increasing those risks. I’m already starting to think of collaborating with RFF colleagues, looking at climate impacts on businesses and how they’re able to handle that risk.

Hannah Druckenmiller
How Much Is a Tree Worth?
Hannah Druckenmiller, who studies the value of healthy ecosystems and the causes of long-run environmental changes, likewise joined RFF as a fellow in August last year. Elaborating on her various research projects on Resources Radio, Druckenmiller described the economic value of trees, based on how tree mortality shapes property values, air quality, wildfire risk, and more. She also described an ongoing project that uses twentieth-century photographs, taken by British aircraft, to approximate modern satellite imagery and estimate changes over time for environmental resources in Africa.
Listen to the Podcast
Daniel Raimi: Let’s start by going all the way back to when you were a kid. When you were young and growing up, were you interested in environmental issues? Did you have experiences with the natural world that were important for you?
Hannah Druckenmiller: Absolutely. I’ve been interested in environmental issues probably for as long as I can remember. I grew up in New York City, but my parents exposed me to the outdoors constantly—probably the place we spent the most time was the beach, so I always loved the ocean. We would go swimming and fishing. Really anything that got me out on the water, I wanted to do.
When I was in high school, I was lucky enough to go to a place called the Island School, which is this really unique school that’s located on an outer island of the Bahamas. The whole concept behind the school was that learning should be experiential—so, instead of learning biology from a textbook, you actually go out and survey the mangrove or snorkel in the coral reefs, and then you come back into the classroom and talk about what you saw and how everything there was interacting.
It ended up being a formative experience for me. It exposed me to all sorts of environmental issues, especially regarding sustainability and how human and natural systems are interwoven, and I think that’s probably the biggest reason why I decided to pursue those topics when I went to college and then to my PhD program.
There’s two tracks that we often find environmental economists have taken. Some of them start with an interest in the environment and then choose economics as a tool to work on that issue. Some folks start by wanting to be an economist and then discovering environmental issues. Which led the way for you—was it the environmental angle or economics?
HD: It was the environmental angle. In college, I started out as an environmental science major, and I had a focus on oceans, so I got to take all these amazing classes in marine biology and ocean chemistry—things like the physics of waves—but I also took some courses in marine resource management, and I got pretty interested in fisheries. I’ve always been interested in oceans. They are so unexplored and unexplained. It’s a unique part of the earth that covers more than 70 percent of surface area, but we have very little idea of what’s happening in most of it.
One of the things I liked the most was that it was at the intersection of a bunch of different disciplines, so you had to understand the underlying biology—but you also had to understand social and political factors to manage these resources effectively. I took a class called World Food Economy at Stanford, which is taught by Roz Naylor. She just so powerfully conveyed to me what a useful tool economics is for understanding how systems work and for effecting change in those systems. That really led me down this path.
One question I want to ask sounds like a really simple question, but it’s really fascinating: How much is a tree worth?
HD: That question is motivated by estimating the social and economic value of healthy forests. A lot of my work is motivated by the idea that we need to be able to quantify the value of natural resources so we can know how to manage them, and so we can weigh the benefits they provide against the costs of environmental protection. I decided to focus on forests because they’re one of our largest sources of natural capital. That’s true in the United States and around the world. We think that forests provide a whole array of ecosystem services, but we don’t have a really good idea of how much they’re worth in terms of dollar value.
Unfortunately, we’ve seen large declines in forest health around the world over the last several decades. Tree mortality rates have doubled in the last 20 years. My paper tries to understand the consequences of those declines in forest health for human well-being.
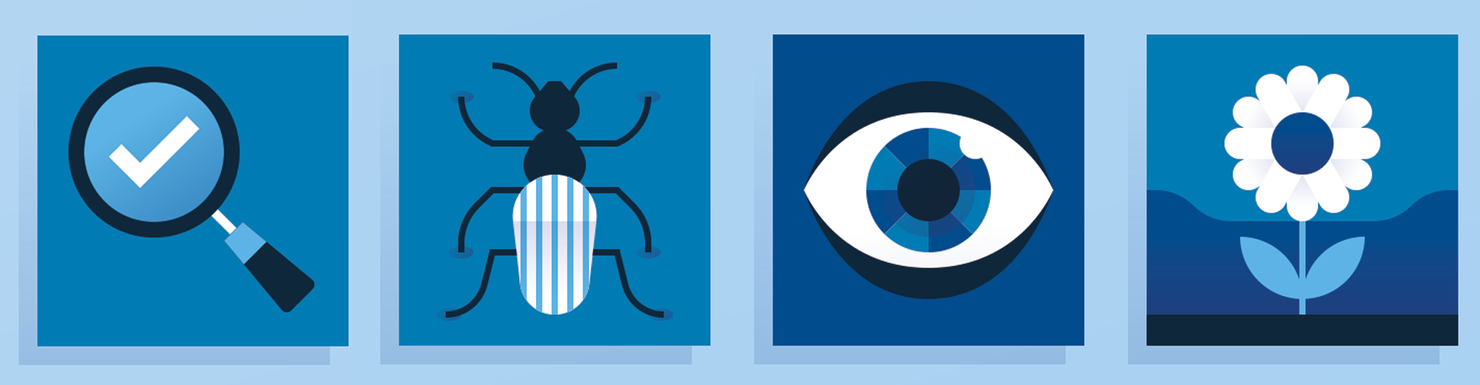
I think, in general, the challenge with valuing natural resources is that many environmental goods and services—including trees—don’t have a clear price in the market. We could go out there and try to estimate the value of a tree by looking at how much timber costs, and that would give us part of the picture—but we know that trees also provide other benefits. They provide aesthetic value and air purification, and healthy trees protect us against floods and fires. We want to capture all those benefits—which in economics, we call nonmarket benefits—when we’re thinking about the total value of a tree. That’s what I try to do in the paper. I try to take into account both the market value of trees and their nonmarket value, so that when we’re thinking about how to manage forests, we can weigh that dollar-value benefit against the cost of investments and forest health.
We’re not going to get into all the details of the methods, but can you give us a thumbnail sketch about how you estimate some of those market and nonmarket benefits?
HD: I can provide a sense of how I measure forest health, which is not straightforward; how I measure economic value; and how I try to create a causal link between those two things.
For forest health, I basically use tree mortality as a summary statistic. I do so for a couple reasons: The first is that we have pretty good data in the United States on tree mortality over time. The Forest Service runs a pretty cool survey where they fly planes over almost all forested areas in the western United States, and they circle areas where they observe dead trees. So, we have these nice annual maps of where tree mortality is occurring and how severe it is. I also chose to focus on tree mortality because it’s a pretty stark indicator of forest health, and it’s been increasing a lot over the last several decades, so it’s something that scientists are interested in understanding the consequences of.
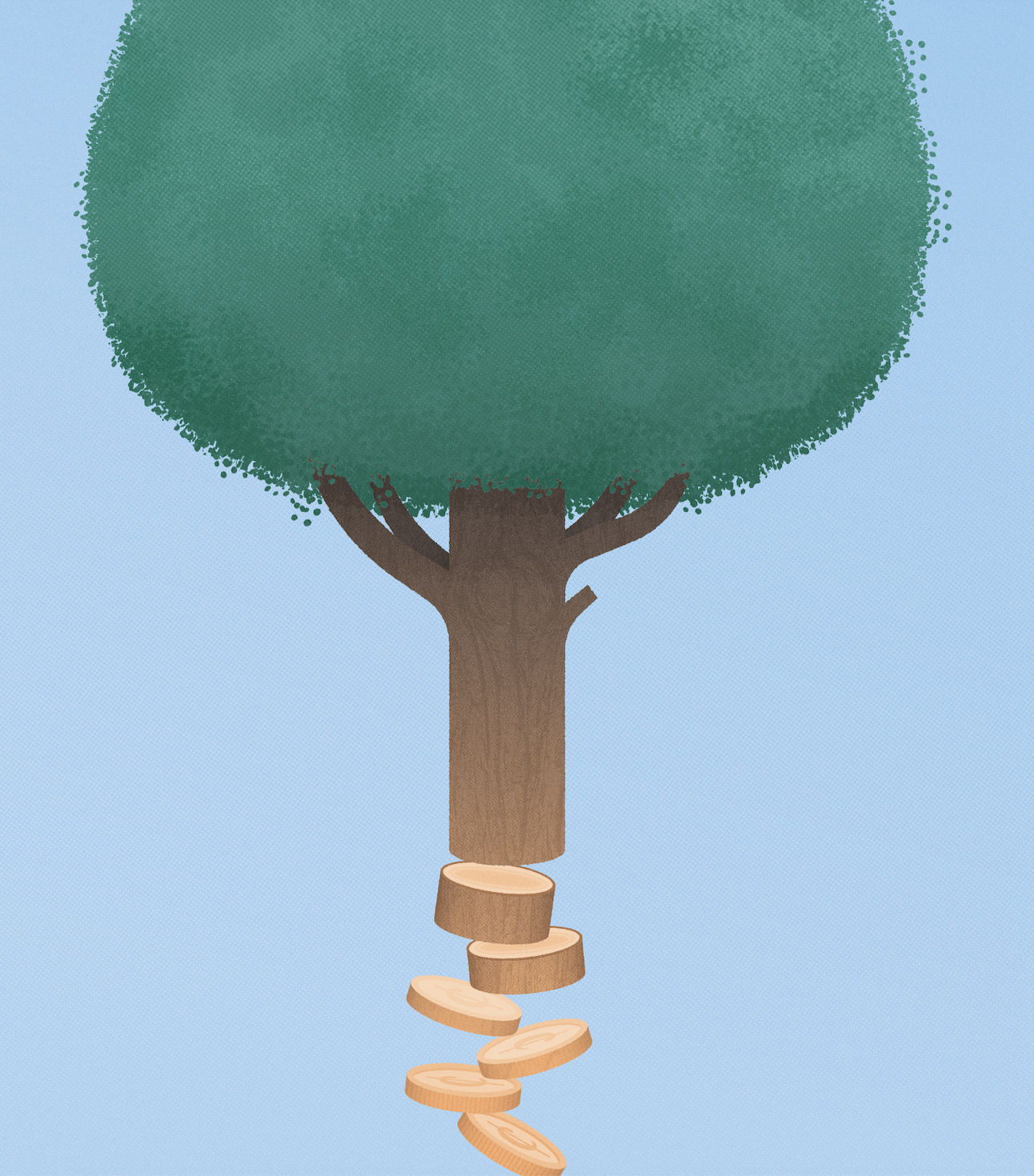
To get at the economic value of trees—again, I’m really focused on capturing both market value and nonmarket benefits. Market value is pretty straightforward, because we can just go out there and see what price people and firms are willing to pay for timber tracts. Nonmarket benefits are more challenging. Luckily, the field of environmental economics has spent a lot of time developing methods to estimate nonmarket benefits, and one of the most popular approaches is called “hedonics,” which is based on the idea that environmental goods and services should capitalize into property values.
You can think of this as the idea that I would probably be more willing to pay more money for a home in an area with lower levels of air pollution, because I value my air quality—similarly, the concept that I would be willing to pay more money for a home in a healthy forest than one in a degraded forest, because I think that healthy trees provide me and my family with some sort of benefits. What we can do is look at the price premium that homeowners are willing to pay for a higher-quality environment, and that’s the dollar value we assign to that resource. That’s what I do in the paper to get at those two different types of benefits.
The last step is to establish a causal link between forest health and the value that we place on trees.
And here comes the beetle.
HD: Yes. We really want that link to be causal, because we’re using this information hopefully to guide policy decisions—we don’t just want a correlation. Which means that we need some sort of random variation in forest health. What I do is I rely on a natural experiment that’s based on bark beetles.
If you’re not familiar with beetles, they’re the leading cause of tree mortality in the American West. They're these tiny bugs that burrow in the bark of trees, and when they breed, they can cause mortality events.
Something that’s really neat about beetles is that their survival is heavily dependent on temperature. In particular, there are temperature thresholds at very low temperatures, where we see mass mortality rates in bark beetles because their tissue freezes. We can look at years that had days just above and below these thresholds—those years are pretty comparable in terms of the rest of the weather distribution—but just one additional day below the thresholds causes large differences in beetle survival and therefore tree mortality. That gives us a way to compare forests that should be similar along many dimensions—but one has very high rates of tree mortality, and one has very low rates of tree mortality.
That’s such a clever way to look at it. What are some of the key results?
HD: Unsurprisingly, I find that beetle population sizes are sensitive to cold temperatures and that tree mortality is very sensitive to beetle survival, so there’s the strong link between very cold days and tree mortality the following summer. I think that’s interesting in its own right, because with climate change, we’re expecting increases in winter temperatures, which would lead to higher rates of beetle survival and higher rates of tree mortality. This is just another thing we need to think about when we’re thinking about managing forests in a changing climate.
The bulk of the paper focuses on understanding the consequences of tree mortality for human well-being. I find that tree mortality greatly reduces the value of timber tracts (the market value of forests), and it also has pretty big impacts on local property values—which, again, are intended to capture some of these nonmarket benefits.
When you add all those things together, I estimate that a tree in my sample is worth about $40—to get at your original question—but it’s worth noting that there’s huge variety in this value across space.
Hannah Druckenmiller
To give you a sense of magnitude, I find that a pretty significant mortality event (you can think of that as like 10 percent of trees in a forest dying) would reduce local property values by 1 to 2 percent and would reduce the value of timber tracts by about $2,500 per acre. These are economically meaningful effects.
I’m also able to look directly at the effect of tree mortality on some specific environmental services. I look at what happens to air quality, wildfire risk, and flood damages when we see mortality events, and I find that tree mortality is actually a strong driver of all three of those natural hazards. This gives us some intuition for why people are willing to pay more for a home in an area with healthier trees, because we have a sense that healthy trees not only provide us with aesthetic value but also might provide us with hazard protection.
When you add all those things together, I estimate that a tree in my sample is worth about $40—to get at your original question—but it’s worth noting that there’s huge variety in this value across space. As you might expect, there’s much higher value for trees that are located in timber-producing regions and for trees that are in areas with high population densities, because more people are exposed to the benefits that those trees provide.
I want to ask you now about another one of your research projects: This one is all about using millions of photographs, taken from aircraft in the middle of the twentieth century of what were then 60 different British colonies, mostly in sub-Saharan Africa. What are you doing with all these pictures taken from airplanes? What information are you trying to gather?
HD: This is a big project that’s a collaboration with researchers at Stockholm University; the University of California, Berkeley; and the National Collection of Aerial Photography in the United Kingdom. The main goal is to extend our understanding of where people were located, where infrastructure was, and where resources were, back in time before data were collected at a large scale or in a systematic way.
Part of the motivation is that the research community has benefited from access to satellite imagery. Starting around 2000, really high-resolution imagery became widely available, and we were able to take that imagery and make it into maps of human development, environmental resources—things that we care about at a global scale. We can look at how deforestation rates have changed over time, or what happens when you add a road to an area, or whether natural resources decline.
This has transformed our understanding of global change. But a big limitation of these data is that they date back only a couple of decades. A lot of the questions that researchers are interested in studying span a longer time period than that. The idea of this project is to try and take advantage of these large archives of aerial photography that were taken over the course of the twentieth century to essentially extend the satellite timeline backward, to the 1940s or ’50s. We like to think of it as providing a window back in time to let us see what was happening on the ground before we had good data collection in a lot of these developing countries.
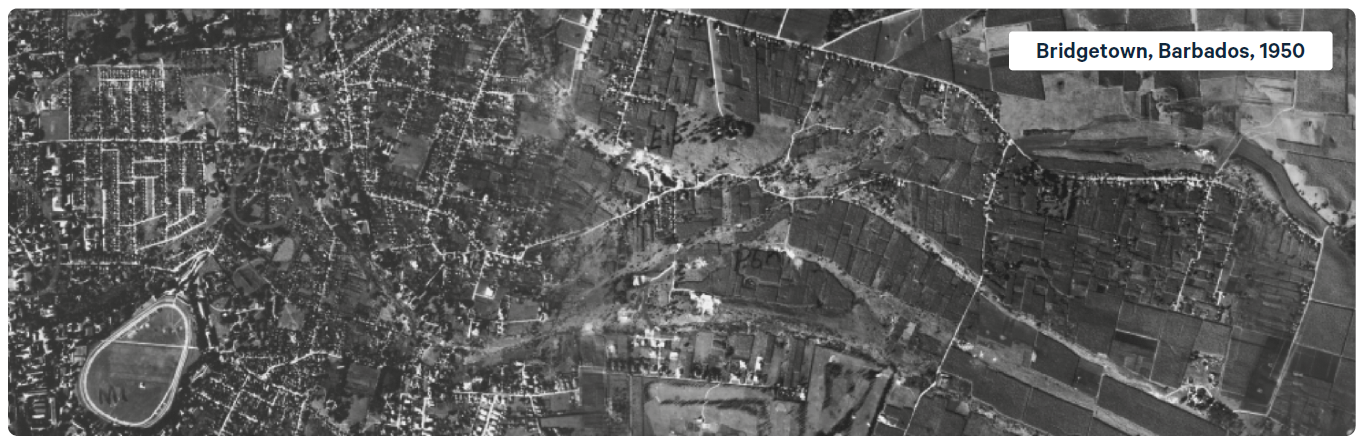
What we’re doing in the project specifically is we have this archive of photos that were taken during the process of mapping the British Empire. The British wanted to understand where people were located, where resources were located, and how these things were changing over time. What we’re doing is taking all the old images and using them to generate data products that map out the location of natural and built capital.
One of the really cool things about the archive is that the countries are visited not only once, but multiple times between 1940 and 1990, so we can create data sets that span different decades and understand how the locations of people and resources were changing over this time period.
It has turned out to be a big undertaking. The photos are in boxes, in the United Kingdom, as physical prints. The first thing we need to do is get them onto our computer—so our partners at the National Collection of Aerial Photography have set up a state-of-the-art scanning operation using robots to digitize the archive at scale. Then, the pictures come to us as black-and-white prints of a location in space—but, unlike a satellite image that you might download, they don’t have any information embedded as to where that image is located in geographic space. That’s something we have to learn from the information contained in the image. We’ve created this whole machine-learning pipeline that essentially takes these millions of individual images and stitches them together into seamless maps—something like you might see if you opened Google Maps and looked at the satellite layer.
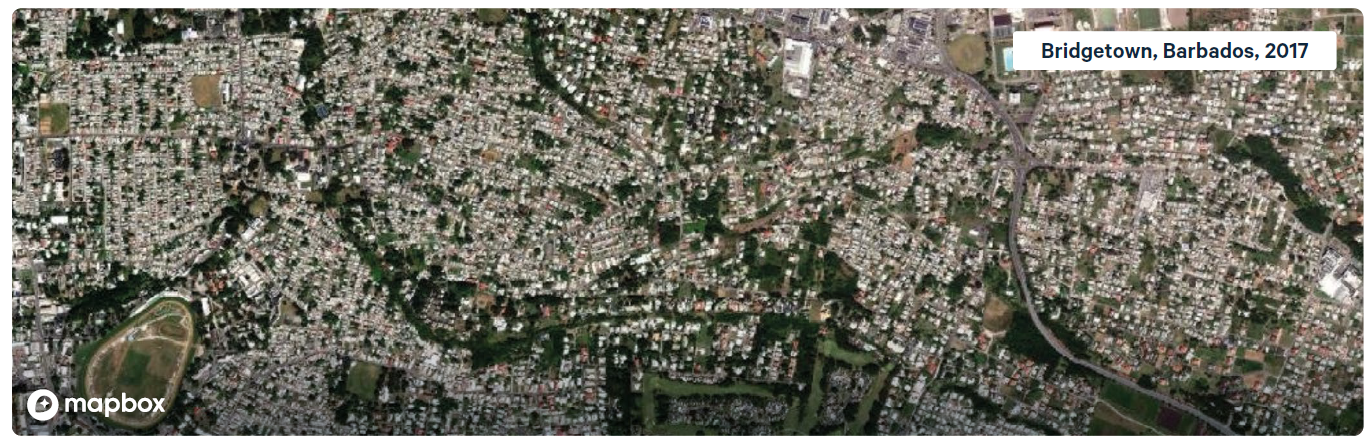
The last step is that we want these images to be helpful to researchers; so instead of just handing them a picture of Kenya in 1950, we want to give them data that they can actually use in their analysis—we need to extract structured information from the imagery. We’re applying out-of-the-box machine-learning tools, like convolutional neural networks, that input the images and output data on road networks or building footprints. We’re making maps of land use, so we can measure forest cover, croplands, and urban extent. These are data sets that we’re lucky enough to currently have access to from about 2000 to 2020, but we think the research community will really benefit from having access to them going much further back in time.
It’s astonishing that you can develop an algorithm to learn where photos were taken without any of that kind of metadata or contextual information. Is it possible to describe how the machine-learning algorithm can ultimately figure out where in space the photo was taken?
HD: There’s still some human input, and we do have some information about where the images are located. What we get is a box full of images—they might be hundreds of images if you’re looking at a small country like Barbados, or thousands of images if you’re looking at a larger country like Kenya. Along with these images, we get a hand-drawn map of where the plane flew. You can think of this as a map of Kenya with a bunch of lines across it that show where the plane was flying—so, we know the order of the images. That allows us to use algorithms that can take two images that have overlap and identify common points between them. I’s more complicated than this with computer vision, but intuitively, it’s like if the computer sees the same road intersection in two adjacent photos, it’s going to align those images so that intersection is overlapping. We do this for every pair of images in the sample.
That’s the intuition of what the computer is doing in this case. It builds us a mosaic of the whole country—and then a person has to place it in geographic space.
Hannah Druckenmiller
But unfortunately, it’s not as easy as that—because if you just lay down one image and then sequentially add images on top of that, these small errors in matching propagate to make something that looks unrecognizable. Our team developed a procedure that essentially optimizes the location of all images jointly, so you can kind of think of this as a person that’s trying to align multiple images into a mosaic on their desk, and you have to shift each image a little bit. When you shift one, you have to shift another so that it matches, and you do that enough times that, finally, you get something that you’re happy with.
That’s the intuition of what the computer is doing in this case. It builds us a mosaic of the whole country—and then a person has to place it in geographic space. They identify points that haven’t changed over time; for example, a coastline or a major highway intersection. By finding just a few of those points, we’re able to locate the entire image on a modern map.
You’ve already hinted at some of the research questions that you or others could answer using these data. But do you have particular applications in mind?
HD: We do hope that the data will be used across a wide range of disciplines; but personally, I’m most interested in understanding the long-term impact of climate shocks. A nice thing about these data is they allow us to look at how impacts persist over time—not just in the next five or ten years, but over half a century. The data also allow us to look at climatic events that happened before we had good information on social and economic outcomes.
We’re applying out-of-the-box machine-learning tools, like convolutional neural networks, that input the images and output data on road networks or building footprints.
Hannah Druckenmiller
One event that I’m really interested in studying is the effect of the Sahel droughts on human migration in Africa. These were decade-long droughts, very severe, that happened between the late 1960s and early 1980s. It’s widely believed that they caused massive famine and displacement of people; but unfortunately, we haven’t been able to study their impact because we haven’t had good data on where people were located during that period.
Climate scientists often think that the Sahel droughts will be a very close analog for the types of droughts that we’ll see under climate change. It would be useful to understand how they affected migrations, so we can use the historical knowledge to inform what we think might happen in the future. But again, we just haven’t had the data to be able to see what it did to populations on the ground.
One thing we can see in these images is human settlement. We plan to pair data on the droughts with newly created data on where people were located and how land was used to try and understand some of the social implications that these droughts had in the 1960s and 1970s.